Growth
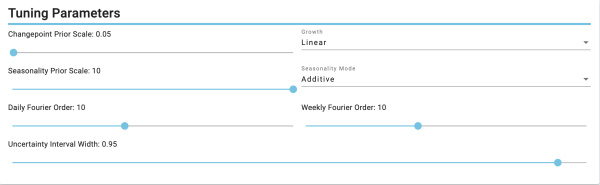
In the context of modeling or forecasting, growth refers to the pattern or trend exhibited by a variable over time. Understanding the concept of growth is essential for analyzing and predicting the behavior of various phenomena, such as sales, population, or resource utilization. When tuning parameters for modeling growth, one crucial consideration is selecting the appropriate growth function, such as linear or flat.
Understanding Growth Functions
Growth functions describe the mathematical relationship between a variable and time. They provide a framework for capturing the underlying trend or pattern exhibited by the data. Two common growth functions are the linear and flat functions:
Linear Growth
A linear growth function assumes a constant rate of change over time. It implies that the variable increases or decreases by a fixed amount in each time period. This function is suitable for situations where the variable exhibits a steady, consistent growth pattern.
Flat Growth
A flat growth function assumes no change over time, indicating that the variable remains constant. This function is appropriate when the variable is expected to remain stable or when there is insufficient evidence to suggest any growth or change.
Selecting a Growth Function
Choosing the appropriate growth function involves considering the nature of the data and the goals of the analysis. The decision depends on several factors:
Data Characteristics
Examine the historical data to identify any noticeable patterns or trends. If there is a consistent upward or downward trajectory, a linear growth function may be suitable. Conversely, if the data appears relatively stable or lacks a discernible trend, a flat growth function might be more appropriate.
Domain Knowledge
Incorporating domain knowledge is crucial in determining the expected growth pattern. Consider the underlying mechanisms or external factors that might influence the variable's behavior. Expert insights or prior research can guide the choice between linear and flat growth functions.
Objective of Analysis
Consider the purpose of the analysis. If the goal is to forecast future values or understand the long-term trajectory, a linear growth function may capture the underlying trend better. On the other hand, if the focus is on short-term stability or comparing different time periods, a flat growth function may be more suitable.
Tuning Parameters
Once the appropriate growth function (linear or flat) has been selected, tuning the associated parameters further refines the model. These parameters control the specifics of the growth function and influence the accuracy of the predictions. The specific parameters may vary depending on the modeling technique used, but some common examples include the slope or growth rate for linear growth and the constant value for flat growth.
Parameter Estimation
The parameters for the chosen growth function are typically estimated using statistical methods such as maximum likelihood estimation or regression techniques. These estimation methods optimize the parameters based on the available data to best fit the chosen growth function.
Validation and Evaluation
It is crucial to validate and evaluate the performance of the chosen growth function and tuned parameters. This can be done by assessing the goodness of fit, conducting out-of-sample validation, or comparing the predicted values against known data points. Iteratively adjusting the parameters based on the evaluation results can improve the model's accuracy.
Conclusion
Understanding growth and selecting the appropriate growth function (linear or flat) are fundamental steps in modeling and forecasting. By considering the nature of the data, domain knowledge, and the analysis objectives, analysts can make informed decisions. Tuning the associated parameters further refines the model, leading to more accurate predictions. Careful consideration of growth and the selection of the appropriate growth function and tuned parameters help ensure meaningful and reliable results in modeling and forecasting scenarios.