Seasonality Mode
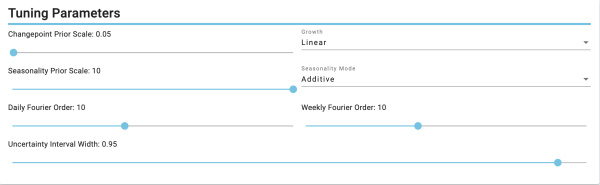
The seasonality mode is a tuning parameter used in time series analysis to model and capture the seasonal patterns present in the data. It determines the relationship between the seasonal component and the overall trend or level of the time series. The two commonly used seasonality modes are additive and multiplicative.
Understanding Seasonal Patterns
Seasonal patterns refer to recurring variations in a time series that follow a specific frequency or time interval. They can be observed in various domains, such as sales data with periodic spikes during holiday seasons or temperature data with regular fluctuations across different seasons. Capturing and modeling these seasonal patterns are crucial for understanding the underlying dynamics of the data.
Key Concepts
To comprehend the role of the seasonality mode, it is important to understand a few key concepts:
Additive Seasonality
In additive seasonality, the seasonal component is assumed to have a constant amplitude or magnitude throughout the time series. The effect of the seasonal pattern is added to the overall trend or level of the data. This implies that the seasonal fluctuations remain relatively consistent regardless of the trend's magnitude.
Multiplicative Seasonality
In multiplicative seasonality, the seasonal component is assumed to have a proportional relationship with the overall trend or level of the time series. The effect of the seasonal pattern is multiplied by the trend. This implies that the seasonal fluctuations increase or decrease proportionally as the trend of the data increases or decreases.
Seasonality Mode
The seasonality mode is a parameter that determines whether the seasonal component should be modeled additively or multiplicatively. It captures the relationship between the seasonal pattern and the overall trend or level of the time series.
Additive Seasonality Mode
Selecting the additive seasonality mode implies that the seasonal component is added to the overall trend or level of the data. It assumes a constant amplitude for the seasonal fluctuations, independent of the trend's magnitude. Additive seasonality is appropriate when the magnitude of the seasonal pattern remains relatively consistent throughout the time series, regardless of the overall trend.
Multiplicative Seasonality Mode
Selecting the multiplicative seasonality mode indicates that the seasonal component is multiplied by the overall trend or level of the data. It assumes a proportional relationship between the seasonal fluctuations and the trend. Multiplicative seasonality is suitable when the magnitude of the seasonal pattern varies proportionally with the overall trend. For example, if the seasonal fluctuations become more pronounced as the trend increases, a multiplicative seasonality mode may be more appropriate.
Tuning the Seasonality Mode
Selecting an appropriate seasonality mode involves understanding the characteristics of the data and considering the relationship between the seasonal pattern and the overall trend or level.
Data Analysis
Examine the behavior of the seasonal patterns in the data. If the magnitude of the seasonal fluctuations appears consistent regardless of the trend's magnitude, the additive seasonality mode may be suitable. Conversely, if the magnitude of the seasonal fluctuations varies proportionally with the trend, the multiplicative seasonality mode may be more appropriate.
Domain Knowledge
Incorporate domain knowledge and expert judgment. Consider any known factors or domain-specific insights about the relationship between the seasonal pattern and the overall trend. Expert insights can guide the selection of an appropriate seasonality mode that aligns with the characteristics of the data and captures the inherent dynamics accurately.
Validation and Evaluation
Validate the selected seasonality mode by evaluating the performance of the time series model. Compare the model's fit to the observed data, assess the accuracy of the forecasts, and examine the residuals. The chosen seasonality mode should provide a satisfactory representation of the seasonal patterns and result in reliable and accurate predictions.
Conclusion
The seasonality mode is a crucial tuning parameter in time series analysis for modeling and capturing seasonal patterns. By selecting the appropriate seasonality mode, either additive or multiplicative, analysts can effectively incorporate the seasonal component into the overall trend or level of the time series.
Choosing between additive and multiplicative seasonality modes depends on the relationship between the seasonal pattern and the trend, as well as the characteristics of the data. The additive seasonality mode assumes a constant amplitude for the seasonal fluctuations, while the multiplicative seasonality mode considers a proportional relationship with the trend.
Consider the behavior of the seasonal patterns in the data and determine whether the magnitude of the seasonal fluctuations remains consistent (additive) or varies proportionally (multiplicative) with the trend. Additionally, domain knowledge and expert judgment play a crucial role in selecting the appropriate mode. Experts familiar with the domain can provide insights into the relationship between the seasonal patterns and the overall trend, guiding the decision-making process.
Validation and evaluation are essential to assess the chosen seasonality mode's performance. Compare the model's fit to the observed data, evaluate the accuracy of the forecasts, and analyze the residuals. The selected seasonality mode should accurately capture the seasonal patterns and improve the model's predictive capabilities.
In conclusion, the seasonality mode is a key tuning parameter for effectively modeling and capturing seasonal patterns in time series analysis. By carefully considering the characteristics of the data and incorporating domain knowledge, analysts can select the appropriate mode—either additive or multiplicative—to accurately represent the relationship between the seasonal component and the overall trend.