Seasonality Prior Scale
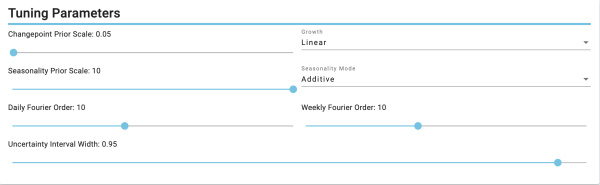
Seasonality is a recurring pattern or cycle in time series data that repeats at regular intervals, such as daily, weekly, or yearly. Capturing seasonality is crucial for accurately modeling and forecasting time series data. The seasonality prior scale is a tuning parameter used in time series analysis algorithms to control the flexibility or smoothness of the estimated seasonal components. It plays a significant role in determining the strength and prominence of the seasonal patterns in the data.
Understanding Seasonality in Time Series Data
Seasonality refers to the regular and predictable fluctuations in a time series that occur due to systematic factors like weather, holidays, or other recurring events. These patterns can have a significant impact on the data and must be properly captured to make accurate predictions and derive meaningful insights.
Key Concepts
To comprehend the role of the seasonality prior scale, it is important to understand a few key concepts:
Seasonal Component
The seasonal component represents the periodic pattern or variation in the data caused by seasonality. It typically exhibits a consistent pattern, such as higher sales during holidays or increased demand during specific months of the year.
Prior Distribution
A prior distribution is a probability distribution that represents our beliefs or uncertainty about the parameters of a statistical model before observing the data. In the context of seasonality, the prior distribution is used to model the strength or prominence of the seasonal component.
Seasonality Prior Scale
The seasonality prior scale is a parameter that controls the flexibility or smoothness of the estimated seasonal components in time series analysis algorithms. It determines the spread or variability of the prior distribution used to model the strength of the seasonal patterns. The scale affects the prominence of the seasonal component in the model.
Large Prior Scale
A larger seasonality prior scale corresponds to a broader prior distribution, allowing for greater variability in the estimated seasonal patterns. This leads to a more flexible model that can accommodate larger fluctuations in the seasonality.
Small Prior Scale
A smaller seasonality prior scale results in a narrower prior distribution, constraining the range of possible seasonal patterns. This leads to a more rigid model with smoother and more regular seasonal components.
Tuning the Seasonality Prior Scale
Selecting an appropriate seasonality prior scale is essential for accurately capturing the seasonal patterns in the data. The optimal scale depends on the specific characteristics of the time series and the desired sensitivity to the seasonal fluctuations.
Trial and Error
Tuning the seasonality prior scale often involves an iterative process. Starting with an initial scale, the analysis is performed, and the results are evaluated. If the estimated seasonal patterns are too flexible or too rigid, the scale can be adjusted accordingly, and the analysis is repeated until satisfactory results are obtained.
Domain Knowledge
Incorporating domain knowledge about the data and the expected magnitude of seasonal variations can guide the selection of an appropriate prior scale. For example, if prior knowledge suggests strong and prominent seasonal patterns, a larger prior scale may be chosen to allow for more variability in the seasonal components.
Comparisons and Validation
It is important to compare the results obtained with different seasonality prior scales and validate them against known or expected seasonal patterns, if available. This helps assess the quality of the estimated seasonality and select the most suitable prior scale.
Conclusion
The seasonality prior scale is a crucial tuning parameter in time series analysis algorithms to capture the recurring patterns in the data. By controlling the flexibility or smoothness of the estimated seasonal components, the prior scale determines the strength and prominence of the seasonal patterns. Selecting an appropriate prior scale involves an iterative process, considering domain knowledge, trial and error, and validation. Through careful tuning of the seasonality prior scale, analysts can accurately capture the underlying seasonality in the data, leading to improved forecasting accuracy and a deeper understanding of the temporal dynamics. It allows for the detection of significant variations in seasonal patterns and helps uncover valuable insights for decision-making, resource allocation, and planning.
It is important to note that the selection of the seasonality prior scale should be guided by the characteristics of the data, the domain expertise, and the specific goals of the analysis. A larger prior scale may be suitable when dealing with data that exhibits strong and pronounced seasonal patterns, while a smaller prior scale may be appropriate for data with more subtle or irregular seasonality.
Validation and evaluation of the estimated seasonal components against known patterns or domain expertise are crucial for assessing the effectiveness of the chosen seasonality prior scale. Additionally, comparing the performance of different scales can help determine the optimal value that strikes a balance between capturing the nuances of the seasonality and avoiding overfitting or underfitting.
In summary, the seasonality prior scale plays a vital role in capturing and modeling the recurring patterns in time series data. By tuning this parameter effectively, analysts can improve the accuracy of their models, enhance forecasting capabilities, and gain valuable insights into the cyclic behavior of the underlying phenomena.